AI in Action: One Student’s Journey to Smarter Sustainability Policy
Posted February 10, 2025
When Ashley Cotsman arrived as a freshman at Georgia Tech, she didn’t know how to code. Now, the fourth-year Public Policy student is leading a research project on AI and decarbonization technologies.
When Cotsman joined the Data Science and Policy Lab as a first-year student, “I had zero skills or knowledge in big data, coding, anything like that,” she said.
But she was enthusiastic about the work. And the lab, led by Associate Professor Omar Asensio in the School of Public Policy, included Ph.D., master’s, and undergraduate students from a variety of degree programs who taught Cotsman how to code on the fly.
She learned how to run simple scripts and web scrapes and assisted with statistical analyses, policy research, writing, and editing. At 19, Cotsman was published for the first time. Now, she’s gone from mentee to mentor and is leading one of the research projects in the lab.
“I feel like I was just this little freshman who had no clue what I was doing, and I blinked, and now I’m conceptualizing a project and coming up with the research design and writing — it’s a very surreal moment,” she said.
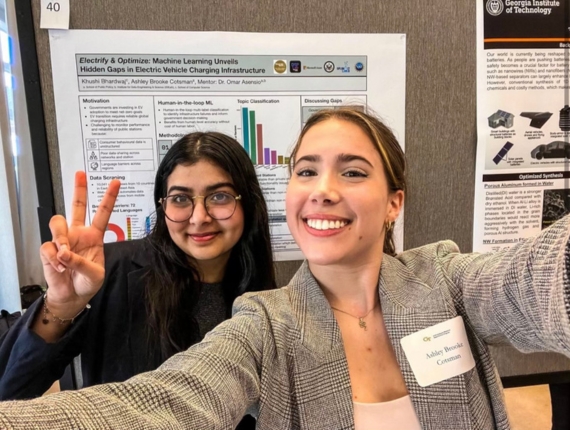
Cotsman, right, presenting a research poster on electric vehicle charging infrastructure, another project she worked on with Asensio and the Data Science and Policy Lab.
What’s the project about?
Cotsman’s project. “Scaling Sustainability Evaluations Through Generative Artificial Intelligence.” uses the large language model GPT-4 to analyze the sea of sustainability reports organizations in every sector publish each year.
The authors, including Celina Scott-Buechler at Stanford University, Lucrezia Nava at University of Exeter, David Reiner at University of Cambridge Judge Business School and Asensio, aim to understand how favorability toward decarbonization technologies vary by industry and over time.
“There are thousands of reports, and they are often long and filled with technical jargon,” Cotsman said. “From a policymaker’s standpoint, it’s difficult to get through. So, we are trying to create a scalable, efficient, and accurate way to quickly read all these reports and get the information.”
How is it done?
The team trained a GPT-4 model to search, analyze, and see trends across 95,000 mentions of specific technologies over 25 years of sustainability reports. What would take someone 80 working days to read and evaluate took the model about eight hours, Cotsman said. And notably, GPT-4 did not require extensive task-specific training data and uniformly applied the same rules to all the data it analyzed, she added.
So, rather than fine-tuning with thousands of human-labeled examples, “it’s more like prompt engineering,” Cotsman said. “Our research demonstrates what logic and safeguards to include in a prompt and the best way to create prompts to get these results.”
The team used chain-of-thought prompting, which guides generative AI systems through each step of its reasoning process with context reasoning, counterexamples, and exceptions, rather than just asking for the answer. They combined this with few-shot learning for misidentified cases, which provides increasingly refined examples for additional guidance, a process the AI community calls “alignment.”
The final prompt included definitions of favorable, neutral, and opposing communications, an example of how each might appear in the text, and an example of how to classify nuanced wording, values, or human principles as well.
It achieved a .86 F1 score, which essentially measures how well the model gets things right on a scale from zero to one. The score is “very high” for a project with essentially zero training data and a specialized dataset, Cotsman said. In contrast, her first project with the group used a large language model called BERT and required 9,000 lines of expert-labeled training data to achieve a similar F1 score.
“It’s wild to me that just two years ago, we spent months and months training these models,” Cotsman said. “We had to annotate all this data and secure dedicated compute nodes or GPUs. It was painstaking. It was expensive. It took so long. And now, two years later, here I am. Just one person with zero training data, able to use these tools in such a scalable, efficient, and accurate way.”
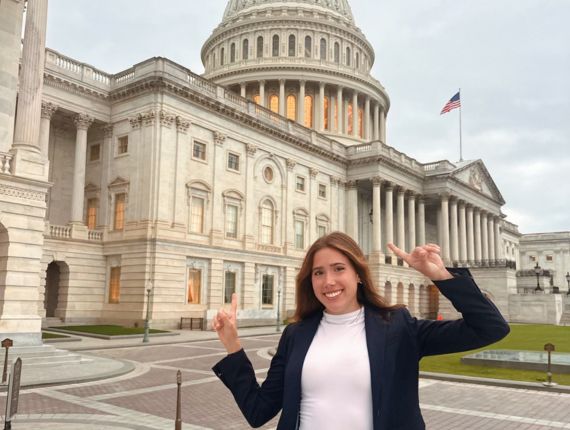
Through the Federal Jackets Fellowship program, Cotsman was able to spend the Fall 2024 semester as a legislative intern in Washington, D.C.
Why does it matter?
While Cotsman’s colleagues focus on the results of the project, she is more interested in the methodology. The prompts can be used for preference learning on any type of “unstructured data,” such as video or social media posts, especially those examining technology adoption for environmental issues. Asensio and the Data Science and Policy team use the technique in many of their recent projects.
“We can very quickly use GPT-4 to read through these things and pull out insights that are difficult to do with traditional coding,” Cotsman said. “Obviously, the results will be interesting on the electrification and carbon side. But what I’ve found so interesting is how we can use these emerging technologies as tools for better policymaking.”
While concerns over the speed of development of AI is justifiable, she said, Cotsman’s research experience at Georgia Tech has given her an optimistic view of the new technology.
“I’ve seen very quickly how, when used for good, these things will transform our world for the better. From the policy standpoint, we’re going to need a lot of regulation. But from the standpoint of academia and research, if we embrace these things and use them for good, I think the opportunities are endless for what we can do.”